Or: Do we still need namedtuple? (spoiler: yes)
Python has several composite types to hold, pass, and iterate over multiple values, and Python functions can take and return multiple arguments.
But there’s still room for something like a struct, a composite type to hold multiple values with a name for each.
Here, I’m comparing some of the better Python “struct” options in their type-hinted forms.
# ----------------------------------------------------------- dictionary
from typing import TypedDict # This only works as of Python 3.8
class ScoreGameDict(TypedDict):
score: float
game: str
my_dictionary: ScoreGameDict = {"score": 3, "game": "piquet"}
# ----------------------------------------------------------- namedtuple
from typing import NamedTuple
ScoreGameNT = NamedTuple("ScoreGameNT", [("score", float), ("game", str)])
my_namedtuple = ScoreGameNT(3, "piquet")
# ---------------------------------------------------- object with slots
class ScoreGameSlots:
__slots__ = ["score", "game"]
def __init__(self, score: float, game: str) -> None:
self.score = score
self.game = game
my_slots = ScoreGameSlots(3, "piquet")
# ------------------------------------------------------------ dataclass
from dataclasses import dataclass
@dataclass
class ScoreGameDC:
score: float
game: str
my_dataclass = ScoreGameDC(3, "piquet")
On to the comparisons.
mutability
- Dictionary is mutable (and extendable)
- Namedtuple is immutable
- Object with slots is mutable (but not extendable)
- Dataclass can be mutable (and extendable) OR immutable (and not extendable)
If you plan to use your struct as an aggregator of data, a slotted object will both allow extension and require (a good thing) you to “register” new keys as you come up with new things to aggregate. You can still make a mess, but it won’t be a hidden mess.
unpacking
Straightforward with namedtuple.
a, b = my_namedtuple
The linter knowns how many values you have to unpack from your namedtuple.
a, b, c = my_namedtuple # ValueError: not enough values to unpack (expected 3, got 2)
With Python 3.7+, you can also unpack a dictionary, as order is guaranteed. However, the linter will not warn you if you try to unpack too many or too few values.
a, b = my_dict.values()
a, b = my_dataclass.__dict__.values()
This isn’t as nice with a slotted object. You’ll have to explicitly iterate through slots, and you still won’t have any help from the linter.
a, b = (getattr(my_slots, x) for x in my_slots.__slots__)
In 3.7+, unpack any user-defined class attributes with vars (except slots). There are pitfalls here. Vars returns a dictionary. The order of items will be consistent, but may not match your intuition, especially if you’ve added attributes in post_init. And, again, no help from the linter.
a, b = vars(my_dataclass).values()
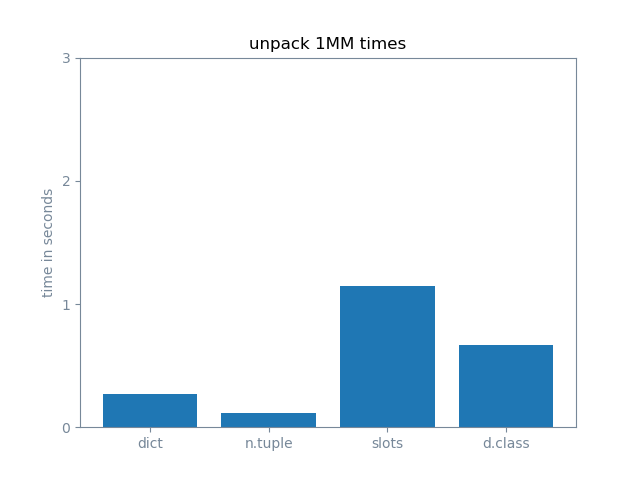
exploding
Exploding each is similar to unpacking.
function_with_kwargs(**my_namedtuple._asdict())
function_with_kwargs(**my_dictionary)
function_with_kwargs(**{x: getattr(my_slots, x) for x in my_slots.__slots__})
function_with_kwargs(**vars(my_dataclass))
Dictionary has an extra trick here. I won’t argue for it, but you *can *sneak illegal attribute names in as kwargs with dictionary exploding. This allows you to pass around e.g. xml or css identifiers.
from xml.etree import ElementTree
def new_line_element(**attributes: str) -> ElementTree.Element:
element = ElementTree.Element("line")
for attribute, value in attributes.items():
element.set(attribute, value)
return element
# pass in illegal Python identifier "stroke-linecap"
new_line_element(x1="0", x2="1", **{"stroke-linecap": "round"})
type hinting
As of Python 3.8, you can now type hint dictionaries per key. This makes all options equivalent in this respect. With older versions of Python, you will not get type hints for dictionaries beyond a union of expected value types.
Dict[str, Union[float, str]]
PyCharm will offer hints when creating namedtuples and the user-defined object types. (And dictionaries as of Python 3.8.)
my_namedtuple = ScoreGameNT( # ... you'd see hint "score: float, game: str"
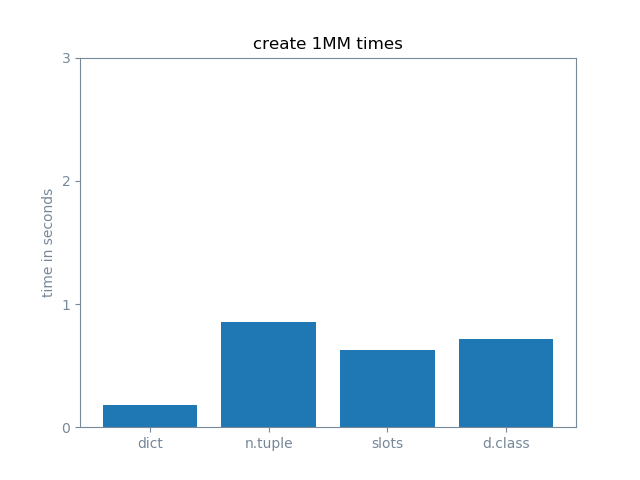
autocomplete / linting
This is a short section, but it may be the most important section in the article.
The linter will let you get away with mis-typed dictionary keys.
a = my_dict["scoer"] # this looks fine till you run it
The linter would pick this up for the other “struct” types.
a = my_namedtuple.scoer # here the linter would start barking
a = my_slots.scoer # here the linter would start barking
a = my_dataclass.scoer # here the linter would start barking
With the extendable types (dictionary and mutable dataclass), you are vulnerable to mis-typing assignments.
my_dict['scoer'] = 4
my_dataclass.scoer = 4
But at least with dataclass, you’ll have autocomplete to help you out.
refactoring
You cannot refactor dictionary keys. Your strings might feel like identifiers, but they are not; they’re just hashable object instances that happen to be human readable.
attribute access
Not much difference here. Namedtuple is a little slower because it’s a tuple underneath. The others are dictionaries underneath. If you had 500 attributes per struct, it would make more of a difference, but I don’t envision anyone’s explicitly naming 500 attributes.
These speed differences are fractions of a second over 1,000,000 iterations.
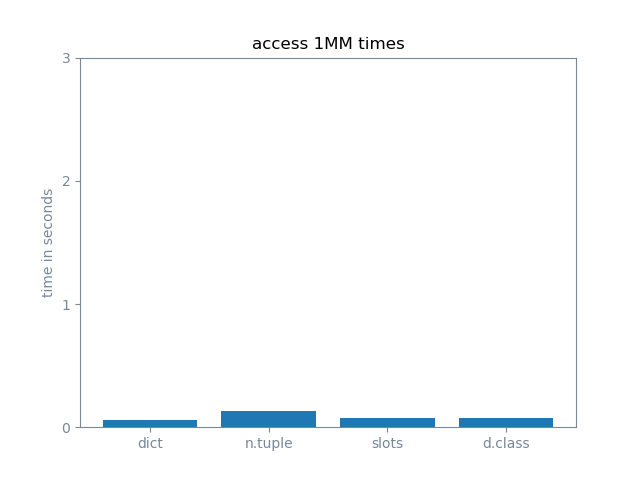
Best of the Python Struct Options?
Named Tuple: Best when you’ll be unpacking and exploding more than creating and accessing, and when your data doesn’t have a more natural mapping. Definitely best when you’re using a named tuple mostly for documentation / standardization purposes and would allow callers to substitute a standard tuple most of the time.
Dictionary: Best when both the values and keys are part of your data (e.g., mapping rows to column headers, employees to positions, etc.).
Slots: Should use less memory than a non-slotted dataclass. That’s its real purpose. As a data container, a slotted object is arguably best when you want to add values over time (safe assignment without __setattr__
hacks and no default values or explicit exclusions required in init).
Dataclass: Best (and fastest) for creating and accessing named data fields with linting, autocomplete, and refactoring. I don’t cover it here, but dataclass has a lot of flexibility with the dataclasses.field
object.